G4 [GEO-SYSTEMS, GEO-MATERIALS, GEO-INFORMATICS, GEO-FLUIDS]
PEER-REVIEWED PUBLICATIONS
* Corresponding Author
- Davydzenka, T., Tahmasebi*, P. and Shokri, N., 2024. Unveiling the global extent of land subsidence: The sinking crisis. Geophysical Research Letters, https://doi.org/10.1029/2023GL104497.
- Hosseini, M.A. and Tahmasebi*, P., 2024. Particle deposition and clogging as an Obstacle and Opportunity for sustainable energy. Journal of Cleaner Production, https://doi.org/10.1016/j.jclepro.2024.141312.
- Hosseini, M.A. and Tahmasebi*, P., 2024. A novel graph-based 3D breakage method for angular particles with an image-based DEM. International Journal of Rock Mechanics and Mining Sciences, 174, https://doi.org/10.1016/j.ijrmms.2024.105640.
- Wu, Y., An, S., Tahmasebi*, P., Liu*, K., Lin, C., Kamrava, S., Liu, C., Yu, C., Zhang, T., Sun, S. and Krevor, S., 2023. An end-to-end approach to predict physical properties of heterogeneous porous media: Coupling deep learning and physics-based features. Fuel, https://doi.org/10.1016/j.fuel.2023.128753.
- Li, P., Liu, M., Alfarraj, M., Tahmasebi, P. and Grana*, D., 2024. Probabilistic physics-informed neural network for seismic petrophysical inversion. Geophysics, https://doi.org/10.1190/geo2023-0214.1.
- Hosseini, M.A. and Tahmasebi*, P., 2023. On the influence of the natural shape of particles in multiphase fluid systems: Granular collapses. Computers and Geotechnics, https://doi.org/10.1016/j.compgeo.2023.105654.
- Mirzaee, H., Kamrava, S. and Tahmasebi*, P., 2023. Minireview on Porous Media and Microstructure Reconstruction Using Machine Learning Techniques: Recent Advances and Outlook. Energy & Fuels, https://doi.org/10.1021/acs.energyfuels.3c02126.
- Wu, Y., Tahmasebi*, P., Liu*, K., Lin, C., Kamrava, S., Liu, S., Fagbemi, S., Liu, C., Chai, R. and An*, S., 2023. Modeling the physical properties of hydrate‐bearing sediments: Considering the effects of occurrence patterns. Energy, https://doi.org/10.1016/j.energy.2023.127674.
- Tahmasebi*, P., 2023. A state-of-the-art review of experimental and computational studies of granular materials: properties, advances, challenges, and future directions. Progress in Materials Science, https://doi.org/10.1016/j.pmatsci.2023.101157.
- Tahmasebi*, P., 2023. Flow in Tight Porous Media. In Album of Porous Media: Structure and Dynamics. Cham: Springer International Publishing. https://doi.org/10.1007/978-3-031-23800-0_104
- Tahmasebi*, P., 2023. Coupling of Pore-Network and Finite Element Methods for Rapid Quantification of Deformation. In Album of Porous Media: Structure and Dynamics. Cham: Springer International Publishing. https://doi.org/10.1007/978-3-031-23800-0_100
- Tao, J., Tahmasebi, P., Kader, M.A., Feng, D., Sahimi, M., Evans, P.D. and Saadatfar*, M., 2023. Wood biomimetics: Capturing and simulating the mesoscale complexity of willow using cross-correlation reconstruction algorithm and 3D printing. Materials & Design, https://doi.org/10.1016/j.matdes.2023.111812.
- Chen, X., Zhao, X., Tahmasebi, P., Luo, C. and Cai*, J., 2023. NMR-data-driven prediction of matrix permeability in sandstone aquifers. Journal of Hydrology, https://doi.org/10.1016/j.jhydrol.2023.129147.
- Chen, X., Thanh, L.D., Luo, C., Tahmasebi, P. and Cai, J., 2023. Dependence of electrical conduction on pore structure in reservoir rocks from Beibuwan and Pearl River Mouth Basins: A theoretical and experimental study. Geophysics, https://doi.org/10.1190/geo2021-0682.1 [PDF]
- Amir Hosseini, M., Kamrava, S., Sahimi, M. and Tahmasebi*, P., (2023). Computer Simulation of the Effect of Wettability on Two-Phase Flow Through Granular Porous Materials. Chemical Engineering Science, https://doi.org/10.1016/j.ces.2023.118446 [PDF]
- Zhang, X., Tahmasebi*, P.: Drafting, Kissing and Tumbling Process of Two Particles: The Effect of Morphology. International Journal of Multiphase Flow. (2023). https://doi.org/10.1016/j.ijmultiphaseflow.2023.104379 [PDF]
- Bai, T. and Tahmasebi*, P., 2022. Graph Neural Network for Groundwater Level Forecasting. Journal of Hydrology. https://doi.org/10.1016/j.jhydrol.2022.128792. [PDF]
- Davydzenka, T., Sinclair, D., Chawla, N. and Tahmasebi*, P., (2022). Deep-layers-assisted machine learning for accurate image segmentation of complex materials. Materials Characterization, https://doi.org/10.1016/j.matchar.2022.112175 [PDF]
- Arora, G., Kamrava, S., Tahmasebi*, P., Adidhy, D. (2022). Charge-density based convolutional neural networks for stacking fault energy prediction in concentrated alloys. Materialia, https://doi.org/10.1016/j.mtla.2022.101620 [PDF]
-
Wu, Y., Tahmasebi*, P., Liu, K., Fagbemi, S., Lin, C., An, S. and Ren, L.: Two-phase flow in heterogeneous porous media: A multiscale digital model approach. International Journal of Heat and Mass Transfer. (2022). https://doi.org/10.1016/j.ijheatmasstransfer.2022.123080 [PDF]
-
Zhang, X., Tahmasebi*, P.: Investigation of particle shape and ambient fluid on sandpiles using a coupled micro-geomechanical model. Powder Technology. (2022). https://doi.org/10.1016/j.powtec.2022.117711 [PDF]
- Sahimi*, M. and Tahmasebi, P., 2022. The Potential of Quantum Computing for Geoscience. Transport in Porous Media, https://doi.org/10.1007/s11242-022-01855-8 [PDF]
-
Ning, Y., Kazemi, H., Tahmasebi, P.: A comparative machine learning study for time series oil production forecasting: ARIMA, LSTM, and Prophet. Computers & Geosciences (2022). https://doi.org/10.1016/j.cageo.2022.105126 [PDF]
-
Bai, T., Tahmasebi*: Characterization of groundwater contamination: A transformer-based deep learning model. Adv. Water Resour. (2022) https://doi.org/10.1016/j.advwatres.2022.104217 [PDF]
-
Davydzenka, T., Tahmasebi*, P. and Carroll, M.: Improving remote sensing classification: A deep-learning-assisted mode. Computers & Geosciences (2022). https://doi.org/10.1016/j.cageo.2022.105123 [PDF]
-
Bai, T., Tahmasebi*, P.: Coupled Hydro-Mechanical Analysis of Seasonal Underground Hydrogen Storage in a Saline Aquifer. Journal of Energy Storage. (2022). https://doi.org/10.1016/j.est.2022.104308 [PDF]
-
Davydzenka, T., Tahmasebi*, P.: High-resolution Fluid-Particle Interactions: A Machine Learning Approach. Journal of Fluid Mechanics. (2022). https://doi.org/110.1017/jfm.2022.174 [PDF]
-
Zhang, X., Tahmasebi*, P.: Coupling irregular particles and fluid: Complex dynamics of granular flows. Computers and Geotechnics. 143, (2019). https://doi.org/10.1016/j.compgeo.2021.104624 [PDF]
-
Poormirzaee, R., Sohrabian, B., Tahmasebi, P.: Seismic refraction data analysis using machine learning and numerical modeling for characterization of dam construction sites. Geophysics 87 (2), U21-U28 (2022). https://doi.org/10.1190/geo2020-0935.1 [PDF]
-
Sahimi*, M., Tahmasebi, P.: Reconstruction, Optimization, and Design of Heterogeneous Materials and Media: Basic Principles, Computational Algorithms, and Applications. Physics Reports (2021) https://doi.org/10.1016/j.physrep.2021.09.003 [PDF]
-
Bai, T., Tahmasebi*, P.: Attention-based LSTM-FCN for Earthquake Detection and Location. Geophysical Journal International (2021). https://doi.org/10.1093/gji/ggab401 [PDF]
-
Kamrava, S., Sahimi*, M., Tahmasebi, P.: Simulating fluid flow in complex porous materials by integrating the governing equations with deep-layered machines. Nat. Comput. Mater. 7, 1–9 (2021). https://doi.org/10.1038/s41524-021-00598-2 [PDF]
-
Karimpouli, S., Tahmasebi, P., Saenger*, E.H.: Ultrasonic prediction of crack density using machine learning: A numerical investigation. Geosci. Front. 101277 (2021). https://doi.org/10.1016/j.gsf.2021.101277 [PDF]
-
Bai, T., Tahmasebi*, P.: Sequential Gaussian simulation for geosystems modeling: A machine learning approach. Geosci. Front. 13, 101258 (2021). https://doi.org/10.1016/j.gsf.2021.101258 [PDF]
-
Kamrava, S., Tahmasebi, P., Sahimi*, M.: Physics- and image-based prediction of fluid flow and transport in complex porous membranes and materials by deep learning. J. Memb. Sci. 622, 119050 (2021). https://doi.org/10.1016/j.memsci.2021.119050 [PDF]
-
Jiang, Z., Tahmasebi*, P., Mao, Z.: Deep residual U-net convolution neural networks with autoregressive strategy for fluid flow predictions in large-scale geosystems. Adv. Water Resour. 150, 103878 (2021). https://doi.org/10.1016/j.advwatres.2021.103878 [PDF]
-
Saenger*, E.H., Finger, C., Karimpouli, S., Tahmasebi, P.: Single-Station Coda Wave Interferometry: A Feasibility Study Using Machine Learning. Materials (Basel). 14, 3451 (2021). https://doi.org/10.3390/ma14133451 [PDF]
-
Bai, T., Jiang, Z., Tahmasebi*, P.: Debris flow prediction with machine learning: smart management of urban systems and infrastructures. Neural Comput. Appl. 1–11 (2021). https://doi.org/0.1007/s00521-021-06197-y [PDF]
-
Tahmasebi, P., Javadpour*, F., Enayati, S.F.: Digital rock techniques to study shale permeability: A mini-review. Energy and Fuels. 34, 15672–15685 (2020). https://doi.org/10.1021/acs.energyfuels.0c03397 [PDF]
-
Bai, T., Tahmasebi*, P.: Accelerating geostatistical modeling using geostatistics-informed machine Learning. Comput. Geosci. 146, 104663 (2021). https://doi.org/10.1016/j.cageo.2020.104663 [PDF]
-
Wu, Y., Tahmasebi*, P., Lin, C., Dong, C.: Using Digital Rock Physics to Investigate the Impacts of Diagenesis Events and Pathways on Rock Properties. J. Pet. Sci. Eng. 108025 (2020). https://doi.org/10.1016/j.petrol.2020.108025 [PDF]
-
Bai, T., Tahmasebi*, P.: Efficient and data-driven prediction of water breakthrough in subsurface systems using deep long short-term memory machine learning. Comput. Geosci. (2020). https://doi.org/10.1007/s10596-020-10005-2 [PDF]
-
Davydzenka, T., Fagbemi, S., Tahmasebi*, P.: Coupled fine-scale modeling of the wettability effects: Deformation and fracturing. Phys. Fluids. 32, 083308 (2020). https://doi.org/10.1063/5.0018455 [PDF]
-
Karimpouli, S., Tahmasebi*, P.: Physics informed machine learning: Seismic wave equation. Geosci. Front. (2020). https://doi.org/10.1016/j.gsf.2020.07.007 [PDF]
-
Fagbemi, S., Tahmasebi*, P., Piri, M.: Elastocapillarity modeling of multiphase flow-induced solid deformation using volume of fluid method. J. Comput. Phys. 421, 109641 (2020). https://doi.org/10.1016/j.jcp.2020.109641 [PDF]
-
Karimpouli, S., Tahmasebi*, P., Ramandi, H.L.: A review of experimental and numerical modeling of digital coalbed methane: Imaging, segmentation, fracture modeling and permeability prediction, (2020). https://doi.org/10.1016/j.coal.2020.103552 [PDF]
- Davydzenka, T., Fagbemi, S., Tahmasebi*, P.: Wettability control on deformation: Coupled multiphase fluid and granular systems. Phys. Rev. E. 102, 013301 (2020). https://doi.org/10.1103/PhysRevE.102.013301 [PDF]
-
Wu, Y., Tahmasebi*, P., Lin, C., Zahid, M.A.M.A., Dong, C., Golab, A.N.A.N., Ren, L.: A comprehensive study on geometric, topological and fractal characterizations of pore systems in low-permeability reservoirs based on SEM, MICP, NMR, and X-ray CT experiments. Mar. Pet. Geol. 103, 12–28 (2019). https://doi.org/10.1016/j.marpetgeo.2019.02.003 [PDF]
-
Fagbemi, S., Tahmasebi*, P.: Coupling pore network and finite element methods for rapid modelling of deformation. J. Fluid Mech. 897, A20 (2020). https://doi.org/10.1017/jfm.2020.381 [PDF]
-
Tahmasebi*, P., Kamrava, S., Bai, T., Sahimi, M.: Machine learning in geo- and environmental sciences: From small to large scale. Adv. Water Resour. 142, 103619 (2020). https://doi.org/10.1016/j.advwatres.2020.103619 [PDF]
-
Bai, T., Tahmasebi*, P.: Hybrid geological modeling: Combining machine learning and multiple-point statistics. Comput. Geosci. 104519 (2020). https://doi.org/10.1016/j.cageo.2020.104519 [PDF]
-
Tahmasebi*, P., Shokri-Kuehni, S.M.S., Sahimi, M., Shokri, N.: How do environmental, economic and health factors influence regional vulnerability to COVID-19? MedRxiv. (2020). https://doi.org/10.1101/2020.04.09.20059659 [PDF]
-
Kamrava, S., Sahimi*, M., Tahmasebi, P.: Quantifying accuracy of stochastic methods of reconstructing complex materials by deep learning. Phys. Rev. E. 101, 043301 (2020). https://doi.org/10.1103/PhysRevE.101.043301 [PDF]
-
Wu, Y., Tahmasebi*, P., Lin, C., Ren, L., Zhang, Y.: Quantitative characterization of non-wetting phase in water-wet porous media based on multiphase flow experiment and numerical simulation. J. Pet. Sci. Eng. 188, (2020). https://doi.org/10.1016/j.petrol.2020.106914 [PDF]
-
Wu, Y., Tahmasebi*, P., Lin, C., Dong, C.: Process-based and dynamic 2D modeling of shale samples: Considering the geology and pore-system evolution. Int. J. Coal Geol. 218, (2020). https://doi.org/10.1016/j.coal.2019.103368 [PDF]
-
Wu, Y., Tahmasebi*, P., Yu, H., Lin, C., Wu, H., Dong, C.: Pore-Scale 3D Dynamic Modeling and Characterization of Shale Samples: Considering the Effects of Thermal Maturation. J. Geophys. Res. Solid Earth. 125, (2020). https://doi.org/10.1029/2019JB018309 [PDF]
-
Fagbemi, S., Tahmasebi*, P., Piri, M.: Numerical modeling of strongly coupled microscale multiphase flow and solid deformation. Int. J. Numer. Anal. Methods Geomech. 44, (2020). https://doi.org/10.1002/nag.2999 [PDF]
-
Kamrava, S., Tahmasebi, P., Sahimi*, M.: Linking Morphology of Porous Media to Their Macroscopic Permeability by Deep Learning. Transp. Porous Media. (2019). https://doi.org/10.1007/s11242-019-01352-5 [PDF]
-
Tahmasebi*, P.: An optimization-based approach for modeling of complex particles. Powder Technol. 356, (2019). https://doi.org/10.1016/j.powtec.2019.08.027 [PDF]
-
Karimpouli*, S., Tahmasebi, P., Saenger, E.H.: Coal Cleat/Fracture Segmentation Using Convolutional Neural Networks. Nat. Resour. Res. (2019). https://doi.org/10.1007/s11053-019-09536-y [PDF]
-
Kamrava, S., Tahmasebi, P., Sahimi*, M.: Enhancing images of shale formations by a hybrid stochastic and deep learning algorithm. Neural Networks. 118, 310–320 (2019). https://doi.org/10.1016/J.NEUNET.2019.07.009 [PDF]
-
Wu, Y., Tahmasebi*, P., Lin, C., Ren, L., Dong, C.: Multiscale modeling of shale samples based on low- and high-resolution images. Mar. Pet. Geol. 109, (2019). https://doi.org/10.1016/j.marpetgeo.2019.06.006 [PDF]
-
Zhang, X., Tahmasebi*, P.: Effects of Grain Size on Deformation in Porous Media. Transp. Porous Media. 129, (2019). https://doi.org/10.1007/s11242-019-01291-1 [PDF]
-
Wu, Y., Tahmasebi*, P., Lin, C., Munawar, M.J., Cnudde, V.: Effects of micropores on geometric, topological and transport properties of pore systems for low-permeability porous media. J. Hydrol. 575, (2019). https://doi.org/10.1016/j.jhydrol.2019.05.014 [PDF]
-
Tahmasebi*, P., Kamrava, S.: A pore-scale mathematical modeling of fluid-particle interactions: Thermo-hydro-mechanical coupling. Int. J. Greenh. Gas Control. 83, (2019). https://doi.org/10.1016/j.ijggc.2018.12.014 [PDF]
-
Mortazavi, M., Tahmasebi*, P., Hezarkhani, A.: Element Mobility in Alteration Zones. Aust. J. Basic Appl. Sci. 4, 197–207 (2010)
-
Karimpouli, S., Tahmasebi*, P.: Segmentation of digital rock images using deep convolutional autoencoder networks. Comput. Geosci. 126, 142–150 (2019). https://doi.org/10.1016/J.CAGEO.2019.02.003 [PDF]
-
Karimpouli, S., Tahmasebi*, P.: Image-based velocity estimation of rock using Convolutional Neural Networks. Neural Networks. 111, 89–97 (2019). https://doi.org/10.1016/J.NEUNET.2018.12.006 [PDF]
-
Fagbemi, S., Tahmasebi*, P., Piri, M.: Interaction Between Fluid and Porous Media with Complex Geometries: A Direct Pore-Scale Study. Water Resour. Res. 54, (2018). https://doi.org/10.1029/2017WR022242 [PDF]
-
Tahmasebi*, P., Kamrava, S.: Rapid multiscale modeling of flow in porous media. Phys. Rev. E. 98, 052901 (2018). https://doi.org/10.1103/PhysRevE.98.052901 [PDF]
-
Fagbemi, S., Tahmasebi*, P., Piri, M.: Pore-scale modeling of multiphase flow through porous media under triaxial stress. Adv. Water Resour. 122, 206–216 (2018). https://doi.org/10.1016/J.ADVWATRES.2018.10.018 [PDF]
-
Tahmasebi*, P., Sahimi, M.: Editorial to the Special Issue on Reconstruction of Porous Media and Materials and Its Applications. Transp. Porous Media. 125, 1–3 (2018). https://doi.org/10.1007/s11242-018-1131-1 [PDF]
-
Karimpouli, S., Tahmasebi*, P.: 3D Multifractal Analysis of Porous Media Using 3D Digital Images: Considerations for heterogeneity evaluation. Geophys. Prospect. 67, 1082–1093 (2019). https://doi.org/10.1111/1365-2478.12681 [PDF]
-
Zhang, X., Tahmasebi*, P.: Micromechanical evaluation of rock and fluid interactions. Int. J. Greenh. Gas Control. 76, 266–277 (2018). https://doi.org/10.1016/J.IJGGC.2018.07.018 [PDF]
-
Tahmasebi*, P., Sahimi, M.: A Stochastic Multiscale Algorithm for Modeling Complex Granular Materials. Granul. Matter. 20, (2018). https://doi.org/10.1007/s10035-018-0816-z [PDF]
-
Tahmasebi*, P.: Packing of discrete and irregular particles. Comput. Geotech. 100, 52–61 (2018). https://doi.org/10.1016/J.COMPGEO.2018.03.011 [PDF]
-
Tahmasebi*, P., Kamrava, S.: A Multiscale Approach for Geologically and Flow Consistent Modeling. Transp. Porous Media. 124, 237–261 (2018). https://doi.org/10.1007/s11242-018-1062-x [PDF]
-
Karimpouli*, S., Tahmasebi, P., Saenger, E.H.: Estimating 3D elastic moduli of rock from 2D thin-section images using differential effective medium theory. GEOPHYSICS. 83, MR211–MR219 (2018). https://doi.org/10.1190/geo2017-0504.1 [PDF]
-
Tahmasebi*, P.: Accurate modeling and evaluation of microstructures in complex materials. Phys. Rev. E. 97, 023307 (2018). https://doi.org/10.1103/PhysRevE.97.023307 [PDF]
-
Tahmasebi*, P., Javadpour, F., Frébourg, G.: Geologic Modeling of Eagle Ford Facies Continuity Based on Outcrop Images and Depositional Processes. SPE J. (2018). https://doi.org/10.2118/189975-PA [PDF]
-
Tahmasebi*, P., Sahimi, M., Shirangi, M.G.: Rapid Learning-Based and Geologically Consistent History Matching. Transp. Porous Media. (2018). https://doi.org/10.1007/s11242-018-1005-6 [PDF]
-
Tahmasebi*, P.: Nanoscale and multiresolution models for shale samples. Fuel. 217, 218–225 (2018). https://doi.org/10.1016/j.fuel.2017.12.107 [PDF]
-
Tahmasebi*, P.: HYPPS: A hybrid geostatistical modeling algorithm for subsurface modeling. Water Resour. Res. 53, 5980–5997 (2017). https://doi.org/10.1002/2017WR021078 [PDF]
-
Tahmasebi*, P., Javadpour, F., Sahimi, M.: Data mining and machine learning for identifying sweet spots in shale reservoirs. Expert Syst. Appl. 88, 435–447 (2017). https://doi.org/10.1016/j.eswa.2017.07.015 [PDF]
-
Karimpouli, S., Tahmasebi*, P., Ramandi, H., Mostaghimi, P., Saadatfar, M.: Stochastic modeling of coal fracture network by direct use of micro-computed tomography images. Int. J. Coal Geol. 179, 153–163 (2017). https://doi.org/10.1016/j.coal.2017.06.002 [PDF]
-
Tahmasebi*, P., Sahimi, M., Andrade, J.E.: Image-based modeling of granular porous media. Geophys. Res. Lett. 44, (2017). https://doi.org/10.1002/2017GL073938 [PDF]
-
Tahmasebi*, P.: Structural adjustment for accurate conditioning in large-scale subsurface systems. Adv. Water Resour. 101, (2017). https://doi.org/10.1016/j.advwatres.2017.01.009 [PDF]
-
Karimpouli, S., Tahmasebi*, P.: A Hierarchical Sampling for Capturing Permeability Trend in Rock Physics. Transp. Porous Media. 116, 1057–1072 (2017). https://doi.org/10.1007/s11242-016-0812-x [PDF]
-
Tahmasebi, P., Sahimi*, M., Kohanpur, A.H., Valocchi, A.: Pore-scale simulation of flow of CO2 and brine in reconstructed and actual 3D rock cores. J. Pet. Sci. Eng. 155, 21–33 (2017). https://doi.org/10.1016/j.petrol.2016.12.031 [PDF]
-
Tahmasebi*, P., Javadpour, F., Sahimi, M.: Stochastic shale permeability matching: Three-dimensional characterization and modeling. Int. J. Coal Geol. 165, 231–242 (2016). https://doi.org/10.1016/j.coal.2016.08.024 [PDF]
-
Karimpouli, S., Tahmasebi*, P.: Conditional reconstruction: An alternative strategy in digital rock physics. Geophysics. 81, D465–D477 (2016). https://doi.org/10.1190/geo2015-0260.1 [PDF]
-
Tahmasebi*, P., Javadpour, F., Sahimi, M., Piri, M.: Multiscale study for stochastic characterization of shale samples. Adv. Water Resour. 89, 91–103 (2016). https://doi.org/10.1016/j.advwatres.2016.01.008 [PDF]
-
Tahmasebi*, P., Sahimi, M.: Enhancing multiple-point geostatistical modeling: 1. Graph theory and pattern adjustment. Water Resour. Res. 52, 2074–2098 (2016). https://doi.org/10.1002/2015WR017806 [PDF]
-
Tahmasebi*, P., Sahimi, M.: Enhancing multiple-point geostatistical modeling: 2. Iterative simulation and multiple distance function. Water Resour. Res. 52, 2099–2122 (2016). https://doi.org/10.1002/2015WR017807 [PDF]
-
Tahmasebi*, P., Javadpour, F., Sahimi, M.: Multiscale and multiresolution modeling of shales and their flow and morphological properties. Sci. Rep. 5, (2015). https://doi.org/10.1038/srep16373 [PDF]
-
Tahmasebi*, P., Javadpour, F., Sahimi, M.: Three-Dimensional Stochastic Characterization of Shale SEM Images. Transp. Porous Media. 110, 521–531 (2015). https://doi.org/10.1007/s11242-015-0570-1 [PDF]
-
Scheidt, C., Tahmasebi, P., Pontiggia, M., Da Pra, A., Caers*, J.: Updating joint uncertainty in trend and depositional scenario for reservoir exploration and early appraisal. Comput. Geosci. 19, 805–820 (2015). https://doi.org/10.1007/s10596-015-9491-x [PDF]
-
Tan, X., Tahmasebi, P., Caers*, J.: Comparing training-image based algorithms using an analysis of distance. Math. Geosci. 46, (2014). https://doi.org/10.1007/s11004-013-9482-1 [PDF]
-
Tahmasebi*, P., Sahimi, M.: Reconstruction of nonstationary disordered materials and media: Watershed transform and cross-correlation function. Phys. Rev. E – Stat. Physics, Plasmas, Fluids, Relat. Interdiscip. Top. 91, 032401 (2015). https://doi.org/10.1103/PhysRevE.91.032401 [PDF]
-
Tahmasebi*, P., Sahimi, M.: Geostatistical Simulation and Reconstruction of Porous Media by a Cross-Correlation Function and Integration of Hard and Soft Data. Transp. Porous Media. 107, 871–905 (2015). https://doi.org/10.1007/s11242-015-0471-3 [PDF]
-
Rezaee, H., Marcotte*, D., Tahmasebi, P., Saucier, A.: Multiple-point geostatistical simulation using enriched pattern databases. Stoch. Environ. Res. Risk Assess. 29, 893–913 (2014). https://doi.org/10.1007/s00477-014-0964-6 [PDF]
-
Hashemi*, S., Javaherian, A., Ataee-pour, M., Tahmasebi, P., Khoshdel, H.: Channel characterization using multiple-point geostatistics, neural network, and modern analogy: A case study from a carbonate reservoir, southwest Iran. J. Appl. Geophys. 111, 47–58 (2014). https://doi.org/10.1016/j.jappgeo.2014.09.015 [PDF]
-
Mahmud*, K., Mariethoz, G., Caers, J., Tahmasebi, P., Baker, A.: Simulation of Earth textures by conditional image quilting. Water Resour. Res. 50, 3088–3107 (2014). https://doi.org/10.1002/2013WR015069 [PDF]
-
Tahmasebi, P., Sahimi, M., Caers*, J.: MS-CCSIM: Accelerating pattern-based geostatistical simulation of categorical variables using a multi-scale search in Fourier space. Comput. Geosci. 67, 75–88 (2014). https://doi.org/10.1016/j.cageo.2014.03.009 [PDF]
-
Tahmasebi, P., Sahimi*, M.: Cross-correlation function for accurate reconstruction of heterogeneous media. Phys. Rev. Lett. 110, 078002 (2013). https://doi.org/10.1103/PhysRevLett.110.078002 [PDF]
-
Tahmasebi, P., Sahimi*, M.: Reconstruction of three-dimensional porous media using a single thin section. Phys. Rev. E – Stat. Nonlinear, Soft Matter Phys. 85, 1–13 (2012). https://doi.org/10.1103/PhysRevE.85.066709 [PDF]
-
Tahmasebi, P., Sahimi*, M., Mariethoz, G., Hezarkhani, A.: Accelerating geostatistical simulations using graphics processing units (GPU). Comput. Geosci. 46, 51–59 (2012). https://doi.org/10.1016/j.cageo.2012.03.028 [PDF]
-
Tahmasebi*, P., Hezarkhani, A.: A fast and independent architecture of artificial neural network for permeability prediction. J. Pet. Sci. Eng. 86–87, 118–126 (2012). https://doi.org/10.1016/j.petrol.2012.03.019 [PDF]
-
Mafakheri, E., Tahmasebi*, P., Ghanbari, D.: Application of artificial neural networks for prediction of coercivity of highly ordered cobalt nanowires synthesized by pulse electrodeposition. Meas. J. Int. Meas. Confed. 45, 1387–1395 (2012). https://doi.org/10.1016/j.measurement.2012.03.027 [PDF]
-
Tahmasebi*, P., Hezarkhani, A., Mortazavi, M.: Application of discriminant analysis for alteration separation. Aust. J. Basic Appl. Sci. 4, (2010)
-
Tahmasebi*, P., Hezarkhani, A.: A hybrid neural networks-fuzzy logic-genetic algorithm. Comput. Geosci. 42, 18–27 (2012). https://doi.org/10.1016/j.cageo.2012.02.004 [PDF]
-
Tahmasebi*, P., Hezarkhani, A.: Application of a Modular Feedforward Neural Network. Nat. Resour. Res. 20, 25–32 (2011). https://doi.org/10.1007/s11053-011-9135-3 [PDF]
-
Tahmasebi*, P., Hezarkhani, A.: Comparison of optimized neural network with fuzzy logic. Aust. J. Basic Appl. Sci. 4, (2010)
-
Tahmasebi*, P., Hezarkhani, A., Sahimi, M.: Multiple-point geostatistical modeling based on the cross-correlation functions. Comput. Geosci. 16, 779–797 (2012). https://doi.org/10.1007/s10596-012-9287-1 [PDF]
-
Asadisaghandi, J., Tahmasebi*, P.: Comparative evaluation of back-propagation neural network learning algorithms and empirical correlations. J. Pet. Sci. Eng. 78, 464–475 (2011). https://doi.org/10.1016/J.PETROL.2011.06.024 [PDF]
-
Tahmasebi*, P., Hezarkhani, A.: Application of adaptive neuro-fuzzy inference system. Aust. J. Basic Appl. Sci. 4, 408–420 (2010)
BOOKS & BOOK CHAPTERS
- Wu, Y. and Tahmasebi*, P., 2023. Digital Rock Modeling: A Review. Physics of Fluid Flow and Transport, 53-76.
-
Tahmasebi, P., 2021, Geotechnics, B. S. Daya Sagar et al. (eds.), Encyclopedia of Mathematical Geosciences, Encyclopedia of Earth Sciences Series (Download)
-
Tahmasebi, P., 2021, Geomechanics: definitions, current issues, and future outlook, B. S. Daya Sagar et al. (eds.), Encyclopedia of Mathematical Geosciences, Encyclopedia of Earth Sciences Series (accepted)
-
Tahmasebi P. Multiple Point Statistics: A Review. Handb. Math. Geosci., Cham: Springer International Publishing; 2018, p. 613–43. https://doi.org/10.1007/978-3-319-78999-6_30. (PDF)
-
Tahmasebi P, Sahimi M. Geostatistical simulation and reconstruction of porous media, Handbook of porous media, 3rd edition, Edited by Kambiz Vafai, CRC Press, Baton Rouge, pp. 869-890.
-
Tahmasebi P, Mariethoz G, Geostatistics Applications in Hydrology. In: Handbook of Engineering Hydrology: Modeling, Climate Change, and Variability, Taylor & Francis, ISBN 9781466552463.
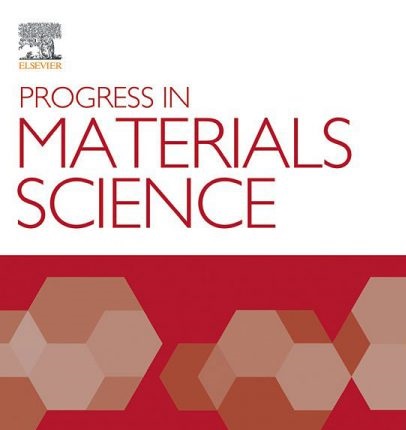

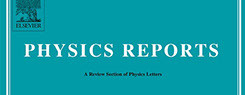
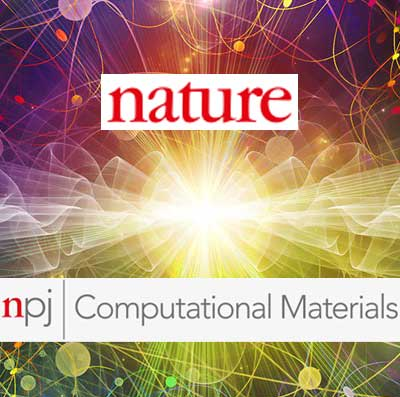
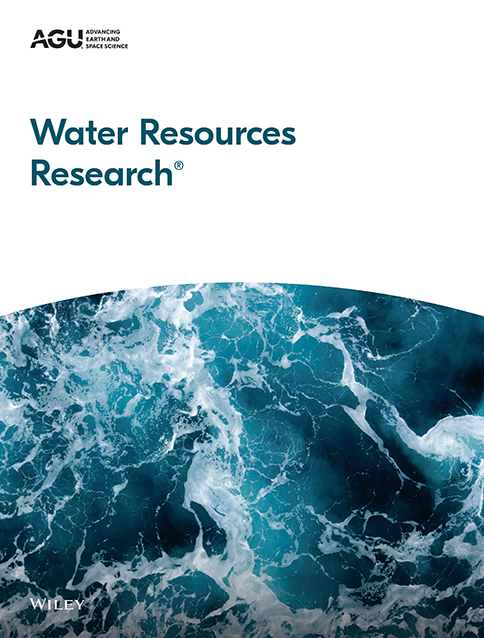


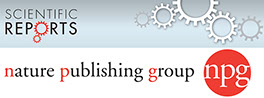
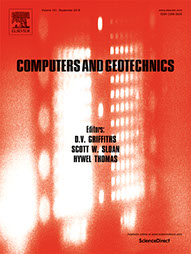

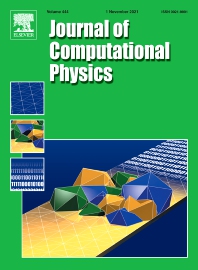







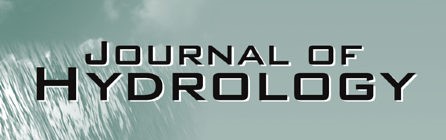
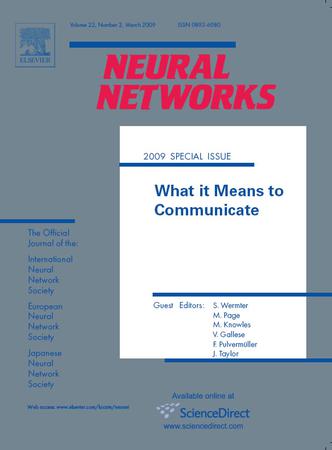